The U.S. Department of Defense’s new ‘Replicator’ effort signals a big, public bet on one principle of war: mass. The program calls for significant investment in the high-volume production of attritable autonomous platforms to overcome problems of access and survivability in a potential conflict with the People’s Republic of China.
If the strategy driving the need for ‘Replicator’ works — if the swarming legions of low-cost systems open windows of opportunity in combat — what should come next? Optimized targeting. Bigger impacts against critical enemy capabilities. Less focus on secondary objectives.
RELATED
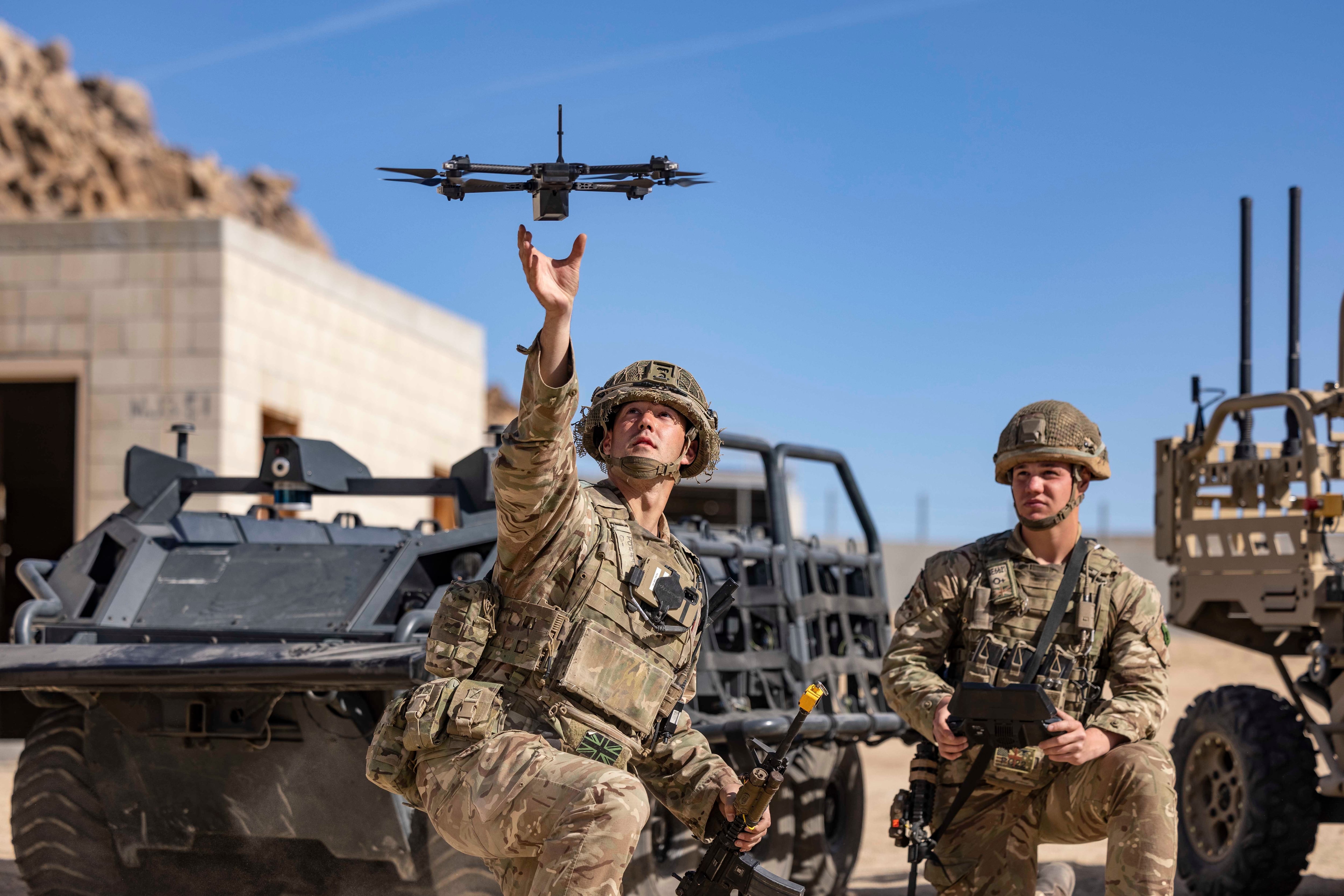
These attributes describe another principle of war: economy of force. And the Pentagon needs to make a big bet on the emerging tech that can enable those attributes. Now. Before it’s too late.
Why now? For starters, I know from experience that there is risk in expending too much combat power on secondary efforts. I led an Air Force unit responsible for identifying and developing targets to counter ISIL. Our team asked leaders for ‘tactical patience’ to deliver high payoff targets, but an appetite amongst some leaders for ever-greater numbers of targets persisted.
The high volume of strikes came at a cost. By 2017 the Department of Defense had dropped so many bombs in Iraq and Syria that it was forced to dip into global reserves to continue the fight from the air. If America ran low on critical weapons against an overmatched adversary like ISIL, are we confident we could sustain a steady barrage of strikes in a protracted conflict against a peer threat?
There was another, greater cost to these strikes. True, the sites we hit were all in use by ISIL at the time; but they were also homes and stores and factories before terrorists occupied them—functions they could no longer serve once the war was over. Mass and persistence can overwhelm an enemy. They can also lead to the devastation of cities and societies for years to come. We can, and should, aim to optimize targeting to achieve desired end states while limiting destruction when possible.
Thankfully, technological advances may soon allow us to achieve these aims in a way previously thought impossible.
Neural networks
Overlooked in the hubbub about autonomy and large language models is meaningful progress in areas that could optimize target selection: probabilistic graphical models and graph neural networks.
Don’t let the big words scare you. If you’ve watched a movie because Netflix recommended it or discovered a new connection on Facebook or benefitted from trendy outfit recommendations on Pinterest, congratulations: you’ve seen these technologies work. They exist to illuminate hidden relationships and identify critical nodes and links in complex networks. They can estimate the probability of success for a course of action given a specified objective. They can help to find signal in noise.
In short: probabilistic graphical models and graph neural networks support the data-driven planning and decision-making that leaders seek in an increasingly volatile and uncertain world.
A look at how we treat bodily infections shows how these technologies could optimize targeting.
Pneumonia can be debilitating for the respiratory system. The resulting sickness is rough: every breath a challenge; every cough painful. If bacteria are the culprit your doctor will likely prescribe antibiotics. These drugs will eliminate your infection, but they will destroy many of the good bacteria in your body, too. They target indiscriminately. The doctor has no choice in this situation. She can’t identify the precise air sacs in the lungs that are under attack, nor can she deliver antibiotics to those nodes alone.
Now imagine your doctor has the capability to map the structure and attributes of each air sac and determine which ones are infected with the help of a graph neural network. And imagine she could use a probabilistic graphical model to simulate many distinct treatment options and provide a tailored, data-driven recommendation for using only enough medication to kill enough bad bacteria so that the infection could no longer sustain itself. This could eliminate your illness while leaving more of your good bacteria intact. The antibiotics saved could treat other patients with separate illnesses. Optimized treatment. Bigger impacts against the harmful agents. Less damage to healthy bacteria.
Doctors can’t yet target only the harmful bacteria with antibiotics but the U.S. military, however, can achieve such precision in hitting its targets. The problem is, and has been, to understand the enemy’s systems with a degree of accuracy and timeliness sufficient to parse the impactful targets from the secondary ones as the enemy adapts and evolves.
Timely, accurate analysis
If developed deliberately and ethically for the purpose of analyzing enemy forces and recommending the most critical targets, probabilistic graphical models and graph neural networks may allow analysts to deliver this timely and accurate analysis to the strategists, planners and operators who require it. But preparing these technologies for military use will require deliberate development. It’s a worthy wager to make in conjunction with that of ‘Replicator’s’ attritable autonomy.
The time to invest is now. At Stanford University, where I am now a visiting scholar, research teams are using these technologies for a range of applications. Nearby Silicon Valley companies are leveraging them to make major breakthroughs on important problems. But these capabilities cannot merely be purchased overnight; most researchers agree that building effective models requires slow, methodical approaches led by well-resourced teams.
If the military wants to leverage and scale these capabilities for tech-assisted optimization of targeting ahead of a potential conflict, the guidance and investment needed to do so must start soon.
The Department of Defense has a deep bench of options for who should head this effort. The Chief Digital and Artificial Intelligence Office recently established a task force to recommend and implement generative AI capabilities across the DoD. Launching a similar task force focused on probabilistic modeling and graph neural networks should be next.
Additionally, the Defense Innovation Unit is uniquely situated near leaders in academic research and commercial applications in Silicon Valley to assess the technical and business use cases of these technologies for battlefield application. Using some of its proposed $1B ‘hedge portfolio’ could help. My favorite: establish another line of effort to test and field these technologies under ‘Replicator’ itself, symbolically harmonizing attritable bulk with optimized precision.
Graph-based models aren’t without problems. Enemy systems are complex, and scaling complexity in graphical models is a challenge. The models need quality data, and implementing an effective data strategy has been a problem for America’s military. Concerns with ‘hallucinations’ in large language models and lack of transparency in how deep-learning models arrive at their conclusions are real and warrant research.
And yet, these challenges and concerns pale in comparison to the risk of losing the first-mover advantage to our adversaries. Or worse, again finding ourselves running low on critical munitions and materiel during combat for lack of an optimized strategy.
Conflict with the People’s Republic of China is neither imminent nor inevitable. But we deter by preparing, and the big bets we make should be balanced. Policymakers and planners must invest now in the technologies that can enable greater optimization of force and firepower in conflict. The recommendation is so clear you don’t even need an AI model to make it for you.
Chance Smith is a U.S. Air Force intelligence officer. He is a visiting scholar at the Center for International Security and Cooperation and the Gordian Knot Center for National Security Innovation, both located at Stanford University. The views expressed are those of the author and do not reflect the official policy or position of the U.S. Air Force, Department of Defense, or the U.S. Government.