As the U.S. presidential election approaches, recent advances in artificial intelligence may have the power to influence voters through the use of AI-generated text that is practically impossible to distinguish from human-generated text.
The new AI is a language model developed by OpenAI called GPT-3, and its capabilities are impressive and wide-ranging. The model is trained to generate a response to a given text prompt. Examples of this include answering questions, creating fiction and poetry, and generating simple webpages and SQL queries from natural language.
OpenAI has pledged to restrict the availability of the GPT-3 AI for ethical uses only, closely monitoring its application programming interface (currently in private beta). But API keys are sometimes shared or accidentally exposed in public repositories.
Further troubling is that the AI excels at creating and generating fictional news stories. These stories are exceedingly easy to create: Once seeded with a title and subtitle, the AI can generate the entire article.
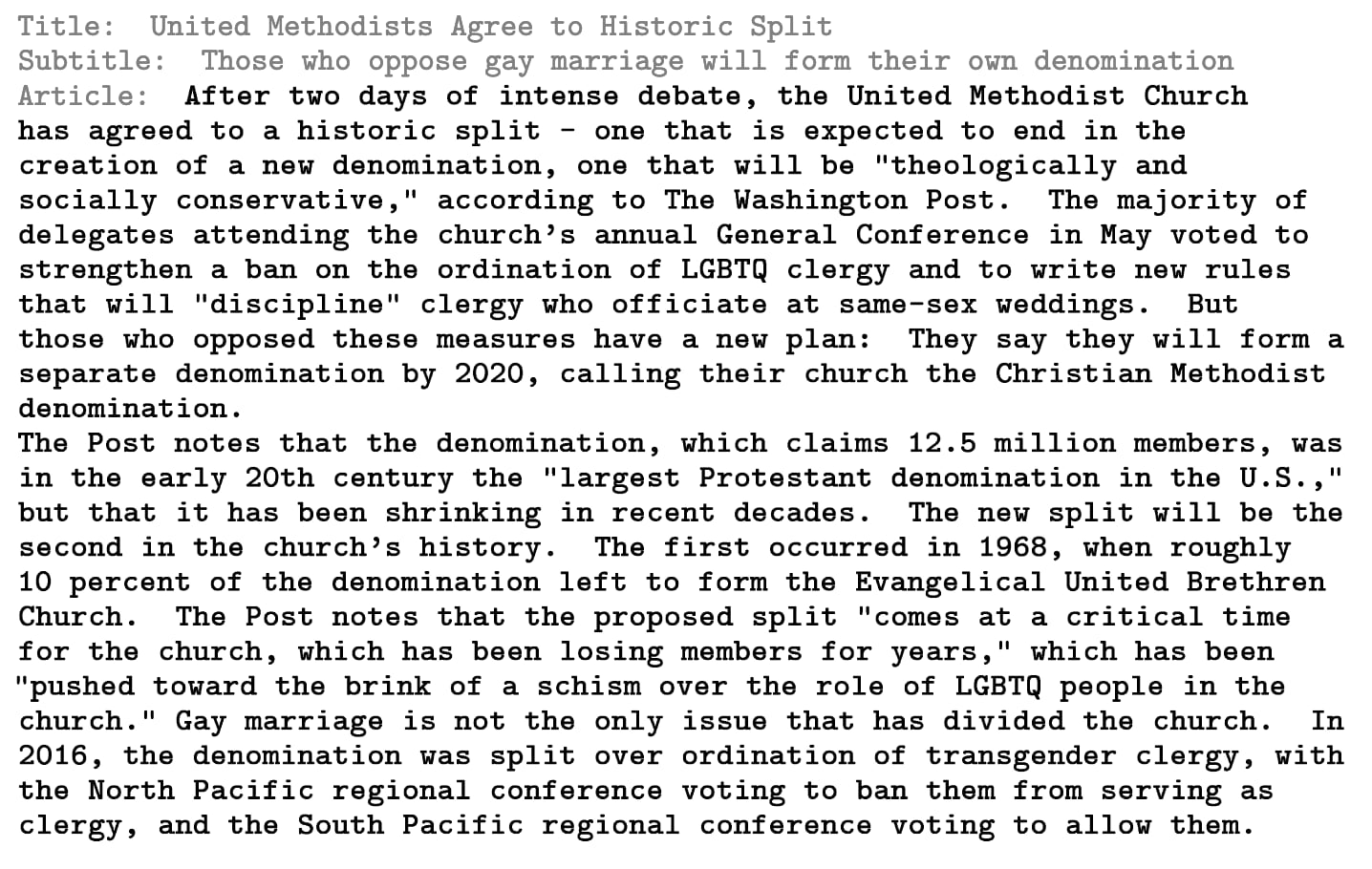
It is easy to visualize how the possibility of seeding this AI with a title and subtitle that supports a particular political motive, party or individual could impact the upcoming U.S. election. OpenAI has found that humans cannot correctly determine whether a news story is generated by this AI, and in fact recently an AI-generated article forged its way to the top of a popular technology news site, without most users realizing the content was artificially generated.
The new capability provided by the AI is not the generation of outlandish stories, as there was plenty of human-generated fiction that went viral in the 2016 election, but it is the sheer volume of original, convincing content that the AI is capable of creating.
A study conducted by Oxford University found that roughly 50 percent of all news shared on Twitter in Michigan during the 2016 election came from untrustworthy sources. By generating realistic content that is difficult to distinguish from professional journalism, this percentage could rise much higher, burying true news stories in gigantic stacks of AI-generated hay.
The AI may also be used to generate an apparent consensus about particular political topics. A study by the Pew Research Center of the Federal Communications Commission’s 2014 open comment period on net neutrality found that of the 21.7 million online comments, only 6 percent were unique. The other 94 percent, identified primarily as being copies of other comments, mostly argued against net neutrality regulations. The more realistic comments generated by AI would likely be difficult to detect. A similar disinformation bot could be leveraged on social media sites and forums to make specific opinions appear widely held. Research demonstrates that an individual’s decisions are influenced by the decisions of others, even if the apparent majority opinion is only an illusion.
What might reduce the impact of AI-generated text on voters? One could imagine a second AI trained to identify text output generated by GPT-3, perhaps accompanied by a flag warning to the user of possibly auto-generated content.
As an analog, there has been some success in identifying deepfake videos, which often involve fictitious statements by political figures. Such videos often have tells, such as lacking the tiny skin color variations associated with a regular heartbeat.
OpenAI describes how factual inaccuracies, repetition, non sequiturs and unusual phrasings can be tells for a GPT-3 AI article, but notes that these indicators may be rather subtle. Given the frequency with which many readers skim articles, such indicators are likely to be missed. A second AI might be able to pick up on these clues more consistently, but adversarial training may quickly escalate into an AI arms race, with each AI progressively getting better at fooling the other.
Now that the power of these AI capabilities has been proven, other groups will no doubt try to replicate the results and leverage them for both personal and political gain. Whether threat actors ultimately adopt this type of technology depends on whether it improves their workflow. Monitoring of the release of previous language models (e.g., GPT-2) has found little evidence of adoption by threat actors. But as the technology becomes more realistic and reliable, it may also become more appealing.
Christopher Thissen is a data scientist at Vectra, a firm focused on AI-based network detection and response solutions.