Americans have watched as AI systems incorrectly matched 28 members of Congress to criminal mugshots, demonstrated bias against women and people of color, and caused a lawyer to inadvertently cite fake cases.
In a recent MITRE-Harris Poll survey, most Americans expressed reservations about AI for high-value applications such as autonomous vehicles, accessing government benefits, and healthcare. Only 48% believe AI is safe and secure, and 78% are very or somewhat concerned that AI can be used for malicious intent. And 82% percent are in favor of government regulation for AI.
RELATED
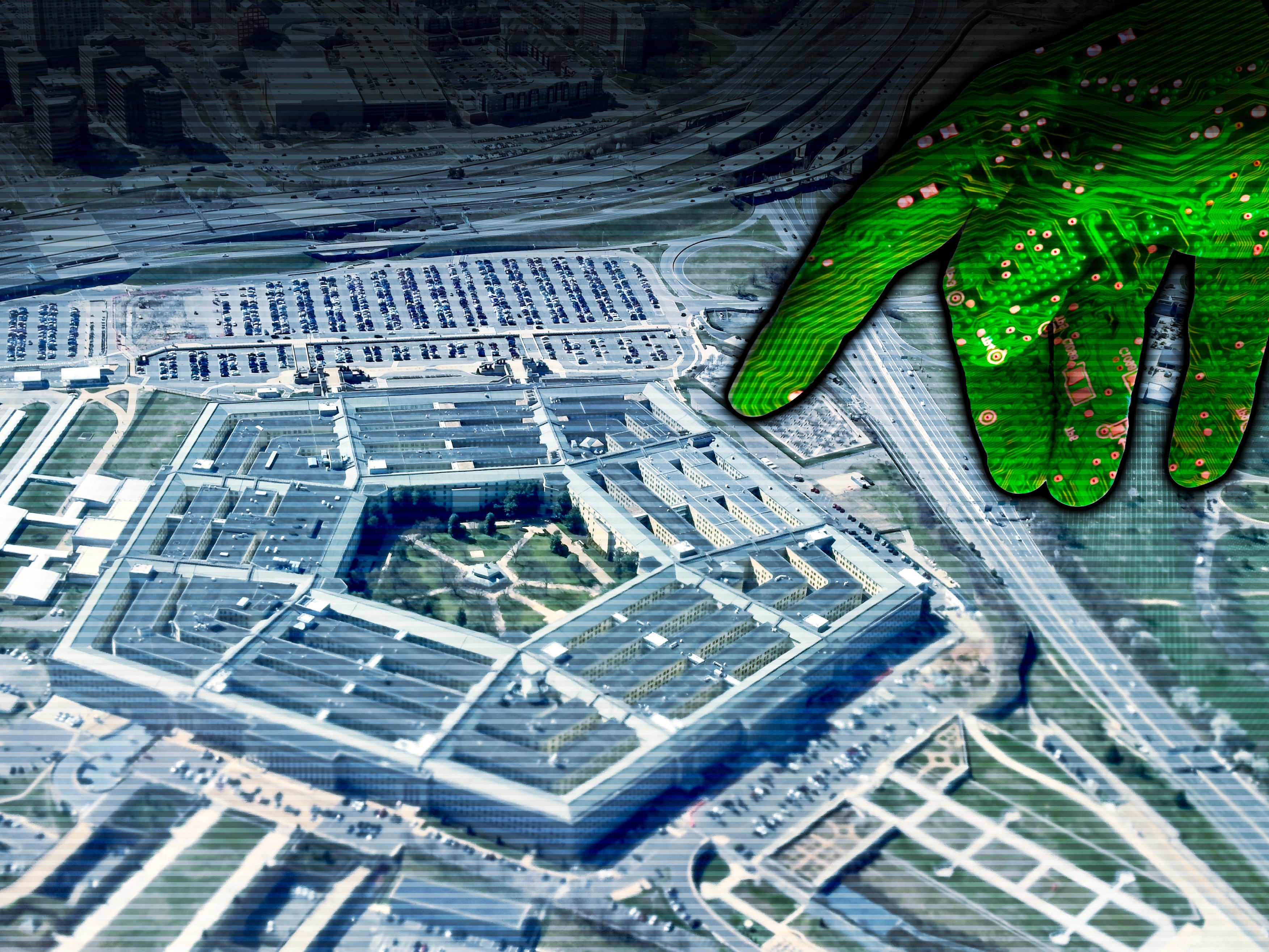
Even Sam Altman, CEO of OpenAI, the company behind ChatGPT, has called for regulation.
The rapid growth of large language models (LLMs) like ChatGPT have changed how people view AI. It has become anthropomorphized and viewed as a standalone entity with its own agency and objectives. This is very different than only a year ago when AI was understood by most to be smart software that lived inside a digital system. When contemplating an approach to AI regulation, it is helpful to treat these two models differently.
For AI as a component within an engineered system, AI assurance–assuring that an AI application does what it’s expected to without unacceptable risks, and in the right context at the right time–is the name of the game. As with any software component, we need testing and validation that verifies things like robustness, security, and correctness. The best equipped to evaluate these attributes are the existing regulators who already have responsibility for relevant industries like healthcare or critical infrastructure. These regulators would be the right group to evaluate risk within the context of their own industry norms.
However, this new domain of LLMs with human-like behaviors and understanding requires a different approach. There are a few scenarios to consider.
First, humans will use AI as a co-pilot to make them more efficient in unwanted or criminal digital behavior, like cyberattacks or misinformation campaigns. While the LLM here is an enabler, the human ultimately remains responsible for their AI-augmented actions in cyberspace. We must ensure we can prevent, defend, remediate, and attribute these actions— much as we do today—but at what will likely become a larger scale.
Second, humans will give agentic AI systems malicious goals. For example, AgentGPT is an instance of GPT with internet access that attempts to execute a high-level task by developing and executing a series of derivative tasks. ChaosGPT is an instance of AgentGPT tasked with exterminating humanity. Fortunately, ChaosGPT has not made much progress in hacking into any national nuclear arsenals, but it represents a hyperbolic example of what may come. Expect criminal ransomware groups to begin using these tools in the near future.
Human accountability
Addressing this scenario, solutions include holding accountable the human who gave the system the malicious goal and continuing to increase the assurance level of critical digital infrastructure to prevent AI-orchestrated network intrusions from being successful. Interfaces where AI is deliberately connected to such systems should also be carefully regulated.
Third, there is considerable concern around AI systems that inadvertently establish their own sub goals that could be unintentionally dangerous. While this may occur, it is just as, if not more likely, that malicious humans will give AI dangerous sub goals. Thus, the solutions to this scenario are the same as the above example.
A few other thoughts on how we can avoid potential dangers with AI technologies without choking innovative research and development:
— Best practices and regulatory standards that apply to traditional software should also extend to AI components. However, it’s important to acknowledge that AI software may introduce unique vulnerabilities that demand assurance measures, including testing standards, standardized code development practices, and rigorous validation frameworks.
— Regulated industries should develop a response plan based on the National Institute of Standards and Technology (NIST) AI Risk Management Framework. Compliance with the NIST framework should be the starting point for identifying potential regulatory approaches in these industries.
— Development of “assurance cases” prior to deployment would provide documented evidence of a system’s compliance with critical assurance properties and behavior boundaries.
— For AI intended to augment human capabilities, regulation should prioritize system transparency and auditability. Holding individuals accountable for intentionally misusing AI to cause harm requires documenting their intent and execution of such intent.
We can complement regulatory efforts with more investment in research to create a common vocabulary and frameworks for AI alignment that will guide future research efforts, ensuring that advancements in AI align with human values and societal well-being. And it’s essential that we invest more in R&D to automatically detect fake content.
The AI revolution is moving so rapidly that we can’t wait long to address these concerns. If we act now, creating the baseline regulations for AI assurance, we can ensure that AI technologies operates in a safe and accountable manner. We need thoughtful regulation that balances innovation with risk mitigation to harness the full potential of AI while safeguarding our society.
T. Charles Clancy is a senior vice president at MITRE, where he serves as general manager for MITRE Labs and chief futurist. This article is adapted from the recently published paper, “A Sensible Regulatory Framework for AI Security.”