Maps are a human construct. They are a medium, an abstraction, through which space is known by how it is represented. Common language in symbols and depictions allow humans to use maps for navigation in places both familiar and previously unvisited. This is a durable skill, and there’s a reason the military still trains navigation by compass and map. But what if artificial intelligence could read and follow maps in the same way?
Published June 13, “Cross-View Policy Learning for Street Navigation” is a report by DeepMind, the UK-based AI company that shares an owner, Alphabet, with Google. More specifically, it’s an evaluation of an experiment to see if AI can be trained using overhead maps to navigate a space, without the extensive route plotting that typically comes with autonomous navigation tools.
“Goal-driven street navigation agents have not so far been able to transfer to unseen areas without extensive retraining, and relying on simulation is not a scalable solution,” wrote the authors.
“Since aerial images are easily and globally accessible, we propose instead to train a multi-modal policy on ground and aerial views, then transfer the ground view policy to unseen (target) parts of the city by utilizing aerial view observations.”
What sets this method apart from how self-driving car navigation algorithms are trained is twofold: it’s the addition of the top-down map as reference point, and it’s the use of entirely new information in the model for navigation. Traditional self-navigation algorithms are run through imagery already mapped, a reserve of scanned data fed into the robot’s brain to teach it how to pilot itself down familiar roads.
“Inspired by the observation that humans can quickly adapt to a new city simply by reading a map, we explore the idea of incorporating comparable top-down visual information into the training procedure of navigation agents, in order to help them generalize to previously unseen streets,” wrote the researchers.
“Instead of using a human-drawn map, we choose aerial imagery, as it is readily available around the world. Moreover, humans can easily do without maps once they become familiar with an environment. This human versatility motivates our work on training flexible RL agents that can perform using both first-person and top-down views.”
To test the new approach, the AI was run through a simulation of an unfamiliar place with the choice to do forward, turn left or right, turn left or right to a greater degree. The AI navigated using panoramic street-view images of the environment, and was sometimes run with the overhead map view. AI with the map view was more successful in the experiment at navigating to where it needed to get than the AI without.
RELATED
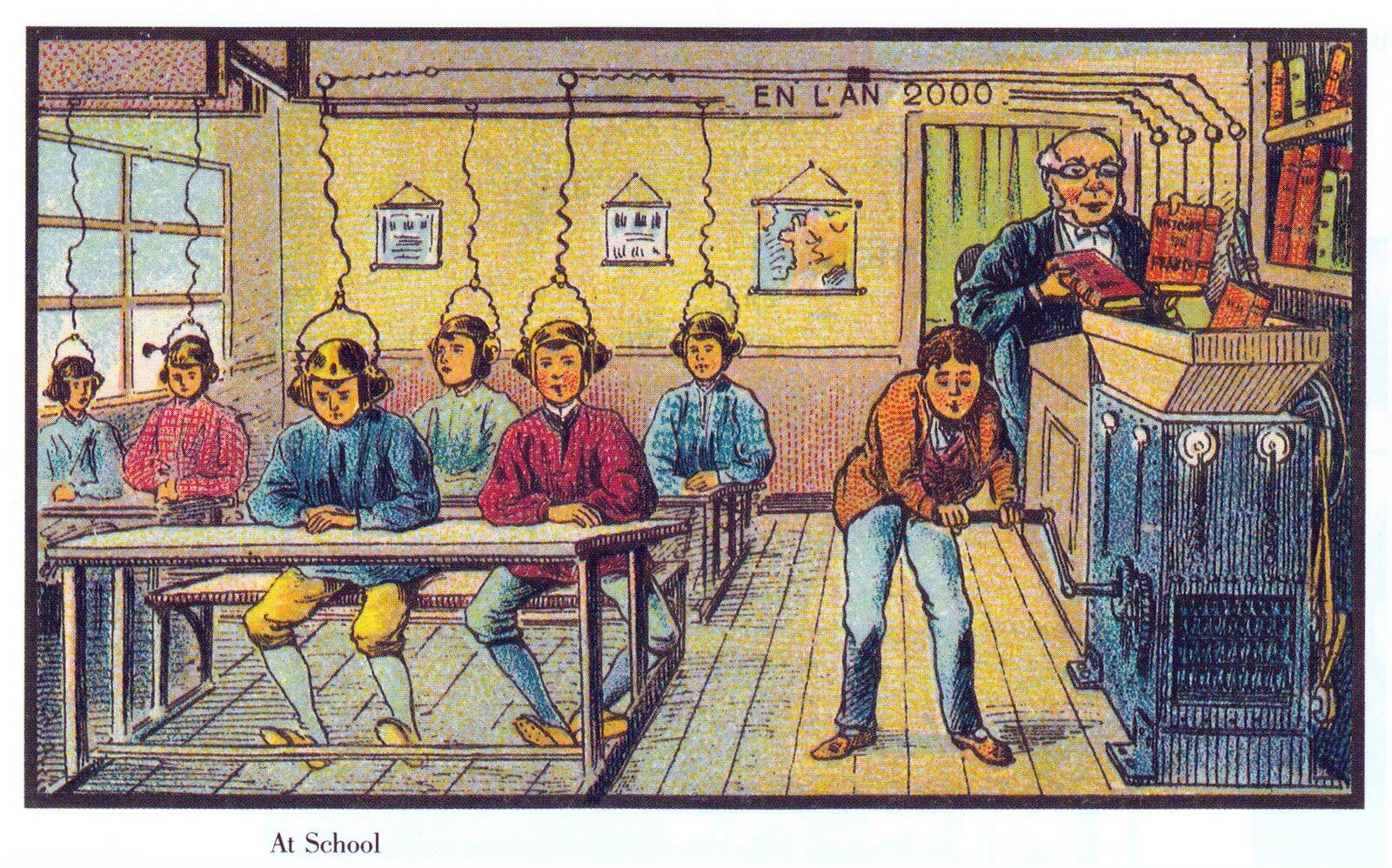
Algorithms like this could be useful for guiding military vehicles that need to travel on lean data in unfamiliar areas. While satellite footage of some quality is available for virtually everywhere on Earth, street-level imagery — especially street-level imagery mapped with LIDAR and iterated in a driving algorithm — is far rarer. Teaching a robot to travel through space based on its own sensors and a map is a lot less of a burden than simulating the exact path beforehand, and matches more the existing capabilities of most remote control and autonomous systems.
It is, in essence, fulfilling the role of the human selecting waypoints on a GPS drop-down, only with the added benefit that the AI navigates on its own and is designed to be independent of GPS. As DARPA looks for AI that can provide military utility on lean data, DeepMind’s map-learning navigation tool could fill a similar need.
The DeepMind researchers open and close their paper with an anecdote about how Ernest Shackleton’s crew used first a map and then, when the map froze, the memory of a map to plot a path to salvation from wreckage. For Shackleton, the map’s memory was a tool to escape the unknown. For the Pentagon, AI that reads maps could be a way to contest the unknown.
Kelsey Atherton blogs about military technology for C4ISRNET, Fifth Domain, Defense News, and Military Times. He previously wrote for Popular Science, and also created, solicited, and edited content for a group blog on political science fiction and international security.